Health IT and the Rise of Personalized Medicine
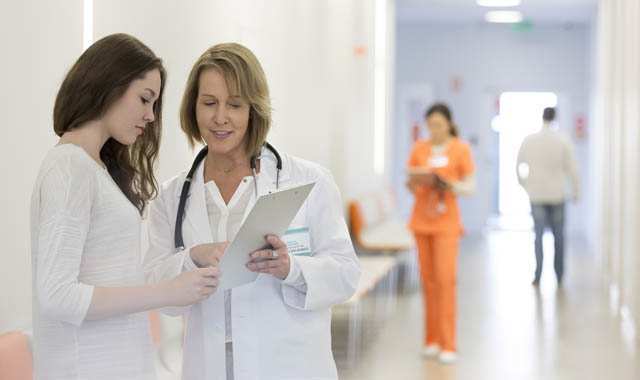
Imagine a cancer treatment tailored to the genetic profile of the patient and the tumor itself. Or an insulin pump that adjusts its dosage using data from body-worn blood sugar and activity monitors. Or a doctor who uses genomic information to choose the right antidepressant for a patient. These are all examples of personalized medicine – and they are happening right now.
In the coming years, we are likely to see hundreds more examples of personalized medicine in action. In large part, this healthcare revolution is being driven by advances in data analytics.
Personalized medicine – also known as precision medicine – is an approach to healthcare that tailors treatments to the individual needs, characteristics and preferences of each patient. Essentially, it is a recognition that all people aren’t the same, so maybe their healthcare shouldn’t be, either.
The precision medicine movement has been enabled in part by advanced in genomics. As we learn more about the human genome, we are able to identify genetic differences that are associated with different outcomes and may indicate different therapeutic approaches. That’s why many women with breast cancer now have genomic assays taken of their tumors in order to identify the best treatment options for their specific type of cancer.
However, personalized medicine isn’t just about genes. It also takes into account environmental and lifestyle factors. For example, a doctor may tailor recommendations for a patient showing signs of metabolic disease to their preferences, time constraints and physical abilities.
This personalized approach to healthcare is made possible by an unprecedented volume of patient data. Patient information may include genomic sequencing data, results of bioassays and other clinical screeners, biometric data from body worn sensors or home health monitoring devices, and behavioral data from electronic logs or mHealth apps. Advanced statistical and analytical methods are needed to turn all of this data into meaningful insights for clinical decision making.
The “big data” approach is helping researchers identify how different patient characteristics (including genetics, environment, lifestyle and compliance) are associated with different treatment outcomes. Not everyone responds to a therapy the same way. While clinical trials are used to demonstrate safety and overall efficacy for a class of patients, they cannot predict how a specific patient will respond. It may take thousands or hundreds of thousands of data points to identify the patterns that allow us to make personalized predictions and treatment recommendations.
We now have a wealth of data available in patient Electronic Health Records (EHRs), including patient characteristics, treatments and outcomes. Researchers can use (anonymized) EHR data, combined with matched genomic data, to identify candidate genes associates with disease development or treatment outcomes. Or they may discover other connections related to non-genetic patient characteristics, lifestyle factors or compliance rates.
Making these connections takes large volumes of data and advanced statistical methods. Battelle has developed an analytical program to help researchers analyze large volumes of medical data. Battelle uses advanced machine learning, pattern recognition and bioinformatics to identify patterns using large volumes of data from disparate sources, including EHR records as well as sensor or imaging data, and genomic data. Finding these patterns and connections will be critical to support more personalized approaches to healthcare.
In the coming years, we are likely to see hundreds more examples of personalized medicine in action. In large part, this healthcare revolution is being driven by advances in data analytics.
Personalized medicine – also known as precision medicine – is an approach to healthcare that tailors treatments to the individual needs, characteristics and preferences of each patient. Essentially, it is a recognition that all people aren’t the same, so maybe their healthcare shouldn’t be, either.
The precision medicine movement has been enabled in part by advanced in genomics. As we learn more about the human genome, we are able to identify genetic differences that are associated with different outcomes and may indicate different therapeutic approaches. That’s why many women with breast cancer now have genomic assays taken of their tumors in order to identify the best treatment options for their specific type of cancer.
However, personalized medicine isn’t just about genes. It also takes into account environmental and lifestyle factors. For example, a doctor may tailor recommendations for a patient showing signs of metabolic disease to their preferences, time constraints and physical abilities.
This personalized approach to healthcare is made possible by an unprecedented volume of patient data. Patient information may include genomic sequencing data, results of bioassays and other clinical screeners, biometric data from body worn sensors or home health monitoring devices, and behavioral data from electronic logs or mHealth apps. Advanced statistical and analytical methods are needed to turn all of this data into meaningful insights for clinical decision making.
The “big data” approach is helping researchers identify how different patient characteristics (including genetics, environment, lifestyle and compliance) are associated with different treatment outcomes. Not everyone responds to a therapy the same way. While clinical trials are used to demonstrate safety and overall efficacy for a class of patients, they cannot predict how a specific patient will respond. It may take thousands or hundreds of thousands of data points to identify the patterns that allow us to make personalized predictions and treatment recommendations.
We now have a wealth of data available in patient Electronic Health Records (EHRs), including patient characteristics, treatments and outcomes. Researchers can use (anonymized) EHR data, combined with matched genomic data, to identify candidate genes associates with disease development or treatment outcomes. Or they may discover other connections related to non-genetic patient characteristics, lifestyle factors or compliance rates.
Making these connections takes large volumes of data and advanced statistical methods. Battelle has developed an analytical program to help researchers analyze large volumes of medical data. Battelle uses advanced machine learning, pattern recognition and bioinformatics to identify patterns using large volumes of data from disparate sources, including EHR records as well as sensor or imaging data, and genomic data. Finding these patterns and connections will be critical to support more personalized approaches to healthcare.
BATTELLE UPDATES
Receive updates from Battelle for an all-access pass to the incredible work of Battelle researchers.